Demand forecasting for products with shorter life cycles presents several challenges due to the rapid changes and uncertainties in the market. Some key challenges include:
- Limited Historical Data: With shorter product life cycles, there is a lack of sufficient historical sales data to accurately predict future demand. This makes it challenging to apply traditional forecasting models that rely on historical patterns and trends.
- Volatility: Markets with shorter product life cycles are often more volatile and subject to sudden shifts in consumer preferences, technological advancements, or competitive landscape. This volatility can lead to inaccuracies in demand forecasts.
- Demand Uncertainty: Forecasting demand for products with short life cycles becomes more uncertain as trends may change quickly, making it difficult to anticipate consumer demand accurately. Factors like marketing campaigns, seasonal fluctuations, or sudden product innovations can impact demand unpredictably.
- Supply Chain Planning: Shorter product life cycles can complicate supply chain planning and inventory management. Forecasting errors can lead to stockouts or excess inventory, affecting both customer satisfaction and company profitability.
- Time Constraints: The compressed timeline of short product life cycles means there is limited time to react to changes in demand forecasts. This requires agile forecasting processes and quick decision-making to adjust production and inventory levels accordingly.
The result of these challenges can be:
- Stockouts: Inaccurate forecasts can lead to underestimating demand, resulting in stockouts and lost sales opportunities.
- Excess inventory: Overestimating demand can leave you with a surplus of unsold products, tying up capital and potentially leading to price reductions.
So, how do businesses cope with these forecasting hurdles? Here are a couple of approaches:
- Advanced analytics: Leveraging techniques like machine learning can help identify subtle patterns in limited data and incorporate external factors into the forecast.
- Analogy-based forecasting: This approach involves identifying similar products with historical sales data to use as a reference for predicting the demand of the new product.
To address these challenges, businesses can leverage advanced analytics, machine learning algorithms, real-time data analytics, and collaborative demand planning with key stakeholders across the supply chain. Additionally, embracing a more agile and responsive approach to demand forecasting can help businesses adapt to the dynamic nature of markets with shorter product life cycles.
Related: What is Demand Forecasting and Why it is Important?
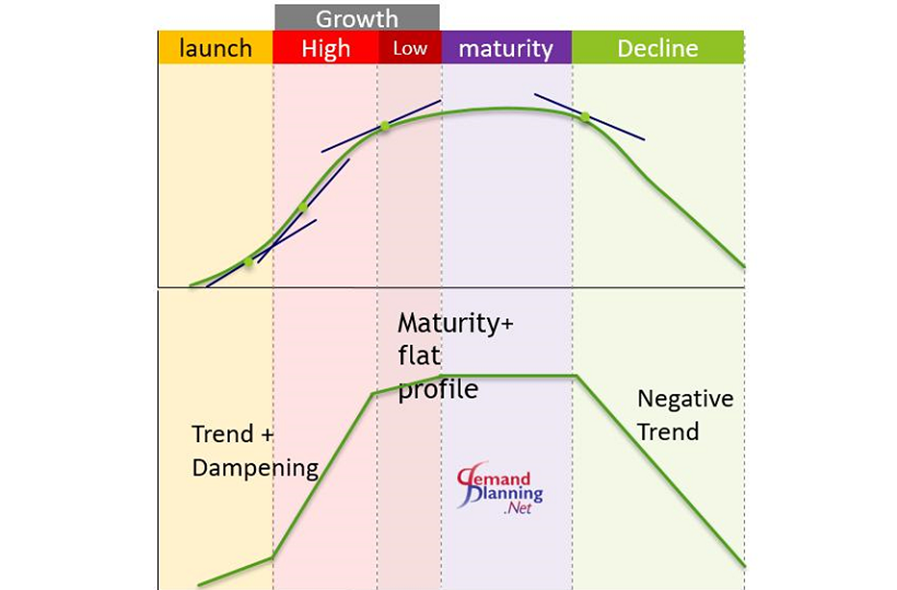
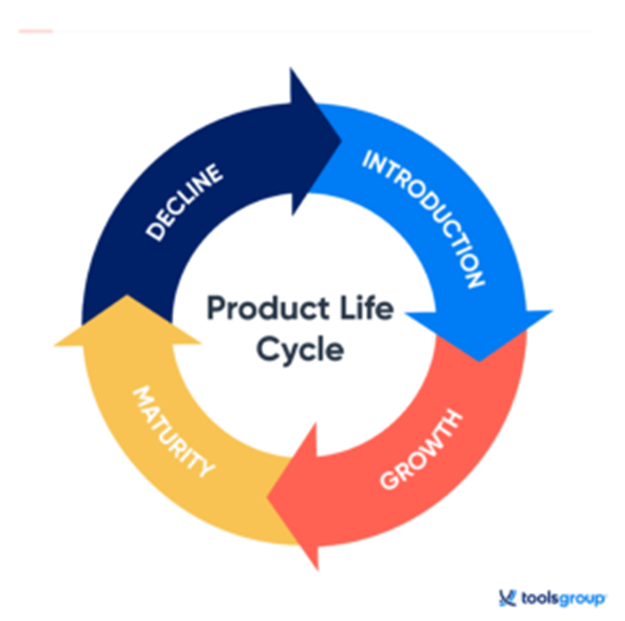
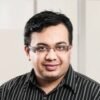
Danish Mairaj is a medical device expert with a strong focus on regulatory and quality compliance. He has been involved in managing clinical trial infrastructure including supplies and logistics. He has over 15 years of experience in the MedTech and Pharmaceutical industry. He is a certified Product Owner, Scrum Master, and Project Management Professional PMP. He studied Biomedical Engineering in Germany and MedTech Regulatory & Quality in Galway, Ireland. He contributes articles to the BRASI newsletter.
- Danish Mairaj#molongui-disabled-link